![]() |
Fonte: Aqui |
09 julho 2019
08 julho 2019
Previsão em Finanças via Machine Learning é muito difícil
[...]
It’s not for lack of interest on Wall Street’s part. The effort to scientifically model markets, which began in the mid-1980s, has absorbed the talents of some of the brightest graduates of math and computer science programs. A handful of secretive hedge fund managers—including Renaissance Technologies, PDT Partners, and D.E. Shaw—have carved out extraordinary returns. But tellingly, many of the leading operations today are the same ones that dominated scientific modeling decades ago. And you probably aren’t rich enough or connected enough to invest with them.
One reason machine investing remains an elite domain is obvious. By definition, most investors can’t beat the average, and every computer that momentarily finds a winning formula will soon face others trying to outwit it. But it turns out that investing is also simply harder than, say, predicting your next Amazon purchase. “It’s one of the most difficult problems in applied machine learning,” says Ciamac Moallemi, a professor at Columbia Business School and a principal at Bourbaki LLC. Here are just some of the devilish problems financial engineers are trying to crack:
The Data Keeps Changing
Or, in quantspeak, it’s nonstationary. An example of stationary data might be the distance between your left eye and your nose. Unless you have plastic surgery, it’s a constant. If a machine is fed hundreds of pictures of you, it will be able to identify you with high probability.
In financial markets, data can change dramatically and in unprecedented ways—for example, when interest rates turned negative across much of Europe and Japan in 2013. Other shifts can be more mundane. In 1998 pricing of U.S. stocks went to decimals from fractions. That wasn’t hard for computers to adjust to, but it might have flustered some of the human traders. “It changed some structure in the market and probably some behavior, too,” says Glen Whitney, a former researcher at Renaissance.
There’s More Noise Than Signal
Stocks move all the time, and not always for any discernible reason. Most market moves are what economists call noise trading. To go back to the image-recognition analogy, imagine a computer trying to identify people in photos that were taken in the dark. Most of the data in those pictures is noise—useless black pixels.
What’s more, as data sets go, the history of stock prices is relatively thin. Say you’re trying to predict how stocks will perform over a one-year horizon. Because we only have decent records back to 1900, there are only 118 nonoverlapping one-year periods to look at in the U.S. Compare this with Facebook Inc., which has an endless trove of stuff to comb through—it processes 350 million pictures a day. And in image recognition, simple tricks such as rotating the photo or altering colors can increase the amount of data; it’s difficult to artificially increase the size of a financial data set.
The Edge You’re Looking for Is Really Small
An obvious signal—for example, to buy stocks on the first day of every month—is not of much use. If that worked in the past, it was probably just a fluke, and even if it isn’t, it’s going to be quickly discovered and traded away by others. So researchers have focused on very faint signals, ones that might predict the future price with only 51% certainty. “We were looking for patterns that are just on the edge of detection,” Whitney says. Most investors can’t take advantage of such patterns. To make them work, money managers have to combine thousands of bets and magnify them with leverage—investing with borrowed money.
Prediction can be improved only so much, forcing elite quantitative managers to look for other advantages. In investing, one profitable problem to solve is transaction costs.
The obvious transaction cost is the fee the broker charges. But there’s also something called slippage, which accounts for the quoted price—$135 for a share of IBM Corp., for example—being relative to the number of shares you want to buy. You might be able to buy only 100 shares at $135; to buy 1,000 shares would require bidding a higher price to attract new sellers. The average cost might then be $136. The only way to know the true price, with slippage, is to transact in the market.
Teaching a machine to anticipate transaction costs helps in two ways. First, the edge required for a trading signal to be profitable might go from 51% to 50.5%. The second advantage is that more can be squeezed from an opportunity. Imagine a widely known model identifies IBM as 1% undervalued. Without understanding transaction costs, a typical company might trade only 1,000 shares, lest it risk too much slippage and push prices above the 1% spread it’s seeking to capture. A company that knows, with perhaps 80% probability, that in fact 5,000 shares can be safely bought without moving the market to higher prices can make a bigger bet. Many in the industry say Renaissance has the most advanced understanding of transaction costs, and that’s one secret to its unequaled track record.
To squeeze transaction costs further, some quant managers build their own high-frequency trading operations, in which they can act as market makers, earning money by matching buyers and sellers. But just as important, running these platforms helps them gain deeper insights into the behavior of the market. It’s akin to Warren Buffett having his own traders on the floor of the New York Stock Exchange rather than using a Wall Street brokerage. Buffett’s own people might tell him things about the mood on the floor that the brokers’ wouldn’t.
Another workaround for quant managers struggling with market data is to find other kinds of information to mine. They’re feeding into their computers everything from satellite photos of parking lots to social media feeds. “Alternative data might be more helpful to firms that are less skilled at wringing signal out of classic data sets,” says Jon McAuliffe, a professor at the University of California at Berkeley and the chief investment officer at Voleon Capital Management LP. Trouble is, such data gets easier and easier to find, so it may not provide an edge for long. (Bloomberg LP, which owns Bloomberg Businessweek, provides clients with access to alternative data.)
Given the complexity of noisy data, most companies try to keep the models as simple as possible. Nick Patterson, who spent a decade as a researcher at Renaissance, says, “One tool that Renaissance uses is linear regression, which a high school student could understand” (OK, a particularly smart high school student; it’s about finding the relationship between two variables). He adds: “It’s simple, but effective if you know how to avoid mistakes just waiting to be made.” Legend holds that at one time the crown jewels of the firm could be written down on a single 8.5-by-11-inch sheet of paper.
As much as hedge funds are using computers for data crunching and pattern recognition, finding new market signals is still a human endeavor. Elite quantitative managers employ huge staffs—sometimes in the hundreds—and show up at machine learning conferences to recruit fresh Ph.D.s.
To build a truly autonomous investing system—one in which the computer itself is thinking about signals and strategies to try—researchers will likely need to crack the problem of causality. That means not only noticing that, for instance, a rise in a particular stock is often accompanied by a bump in interest rates, but also being able to come up with a reason for it. Humans are good at this kind of thinking, but AI has only started to make progress.
Another method, known as deep learning, has driven recent advances in AI, such as image recognition and speech translation. Researchers are tying to bring it to finance, though its use is still limited. Zack Lipton, a professor at Carnegie Mellon, has co-authored a paper showing one possible approach. It addresses the noise problem by predicting not stock prices, but the changes in company fundamentals—such as revenue or profit margins—that ultimately drive returns.
The adversarial nature of trading means that most developments remain shrouded in secrecy. That makes high-quality AI scientists hard to recruit. Scientists like to publish and collaborate. “We love discovering new things about markets and have a great community of people within the firm that we’re able to share results with, but unfortunately we can’t communicate them to a wider audience,” says Pete Muller, the founder of PDT Partners LLC and a pioneer in the field.
The prospect of searching for ghostly signals that eventually disappear can also dissuade some people from working in finance. “In my mind, a top researcher would need a two- to five-times salary multiple to completely forgo the ability to publish and make the lifestyle trade-offs necessary to work in finance,” Lipton says. Still, there’s the lure of a tough problem, combined with the chance to make serious money. “Using machines to beat the markets is a really difficult challenge,” says McAuliffe, whose résumé includes biological research and a stint at Amazon.com Inc. “But I don’t think it’s impossible.”
Fonte: aqui
It’s not for lack of interest on Wall Street’s part. The effort to scientifically model markets, which began in the mid-1980s, has absorbed the talents of some of the brightest graduates of math and computer science programs. A handful of secretive hedge fund managers—including Renaissance Technologies, PDT Partners, and D.E. Shaw—have carved out extraordinary returns. But tellingly, many of the leading operations today are the same ones that dominated scientific modeling decades ago. And you probably aren’t rich enough or connected enough to invest with them.
One reason machine investing remains an elite domain is obvious. By definition, most investors can’t beat the average, and every computer that momentarily finds a winning formula will soon face others trying to outwit it. But it turns out that investing is also simply harder than, say, predicting your next Amazon purchase. “It’s one of the most difficult problems in applied machine learning,” says Ciamac Moallemi, a professor at Columbia Business School and a principal at Bourbaki LLC. Here are just some of the devilish problems financial engineers are trying to crack:
The Data Keeps Changing
Or, in quantspeak, it’s nonstationary. An example of stationary data might be the distance between your left eye and your nose. Unless you have plastic surgery, it’s a constant. If a machine is fed hundreds of pictures of you, it will be able to identify you with high probability.
In financial markets, data can change dramatically and in unprecedented ways—for example, when interest rates turned negative across much of Europe and Japan in 2013. Other shifts can be more mundane. In 1998 pricing of U.S. stocks went to decimals from fractions. That wasn’t hard for computers to adjust to, but it might have flustered some of the human traders. “It changed some structure in the market and probably some behavior, too,” says Glen Whitney, a former researcher at Renaissance.
There’s More Noise Than Signal
Stocks move all the time, and not always for any discernible reason. Most market moves are what economists call noise trading. To go back to the image-recognition analogy, imagine a computer trying to identify people in photos that were taken in the dark. Most of the data in those pictures is noise—useless black pixels.
What’s more, as data sets go, the history of stock prices is relatively thin. Say you’re trying to predict how stocks will perform over a one-year horizon. Because we only have decent records back to 1900, there are only 118 nonoverlapping one-year periods to look at in the U.S. Compare this with Facebook Inc., which has an endless trove of stuff to comb through—it processes 350 million pictures a day. And in image recognition, simple tricks such as rotating the photo or altering colors can increase the amount of data; it’s difficult to artificially increase the size of a financial data set.
The Edge You’re Looking for Is Really Small
An obvious signal—for example, to buy stocks on the first day of every month—is not of much use. If that worked in the past, it was probably just a fluke, and even if it isn’t, it’s going to be quickly discovered and traded away by others. So researchers have focused on very faint signals, ones that might predict the future price with only 51% certainty. “We were looking for patterns that are just on the edge of detection,” Whitney says. Most investors can’t take advantage of such patterns. To make them work, money managers have to combine thousands of bets and magnify them with leverage—investing with borrowed money.
Prediction can be improved only so much, forcing elite quantitative managers to look for other advantages. In investing, one profitable problem to solve is transaction costs.
The obvious transaction cost is the fee the broker charges. But there’s also something called slippage, which accounts for the quoted price—$135 for a share of IBM Corp., for example—being relative to the number of shares you want to buy. You might be able to buy only 100 shares at $135; to buy 1,000 shares would require bidding a higher price to attract new sellers. The average cost might then be $136. The only way to know the true price, with slippage, is to transact in the market.
Teaching a machine to anticipate transaction costs helps in two ways. First, the edge required for a trading signal to be profitable might go from 51% to 50.5%. The second advantage is that more can be squeezed from an opportunity. Imagine a widely known model identifies IBM as 1% undervalued. Without understanding transaction costs, a typical company might trade only 1,000 shares, lest it risk too much slippage and push prices above the 1% spread it’s seeking to capture. A company that knows, with perhaps 80% probability, that in fact 5,000 shares can be safely bought without moving the market to higher prices can make a bigger bet. Many in the industry say Renaissance has the most advanced understanding of transaction costs, and that’s one secret to its unequaled track record.
To squeeze transaction costs further, some quant managers build their own high-frequency trading operations, in which they can act as market makers, earning money by matching buyers and sellers. But just as important, running these platforms helps them gain deeper insights into the behavior of the market. It’s akin to Warren Buffett having his own traders on the floor of the New York Stock Exchange rather than using a Wall Street brokerage. Buffett’s own people might tell him things about the mood on the floor that the brokers’ wouldn’t.
Another workaround for quant managers struggling with market data is to find other kinds of information to mine. They’re feeding into their computers everything from satellite photos of parking lots to social media feeds. “Alternative data might be more helpful to firms that are less skilled at wringing signal out of classic data sets,” says Jon McAuliffe, a professor at the University of California at Berkeley and the chief investment officer at Voleon Capital Management LP. Trouble is, such data gets easier and easier to find, so it may not provide an edge for long. (Bloomberg LP, which owns Bloomberg Businessweek, provides clients with access to alternative data.)
Given the complexity of noisy data, most companies try to keep the models as simple as possible. Nick Patterson, who spent a decade as a researcher at Renaissance, says, “One tool that Renaissance uses is linear regression, which a high school student could understand” (OK, a particularly smart high school student; it’s about finding the relationship between two variables). He adds: “It’s simple, but effective if you know how to avoid mistakes just waiting to be made.” Legend holds that at one time the crown jewels of the firm could be written down on a single 8.5-by-11-inch sheet of paper.
As much as hedge funds are using computers for data crunching and pattern recognition, finding new market signals is still a human endeavor. Elite quantitative managers employ huge staffs—sometimes in the hundreds—and show up at machine learning conferences to recruit fresh Ph.D.s.
To build a truly autonomous investing system—one in which the computer itself is thinking about signals and strategies to try—researchers will likely need to crack the problem of causality. That means not only noticing that, for instance, a rise in a particular stock is often accompanied by a bump in interest rates, but also being able to come up with a reason for it. Humans are good at this kind of thinking, but AI has only started to make progress.
Another method, known as deep learning, has driven recent advances in AI, such as image recognition and speech translation. Researchers are tying to bring it to finance, though its use is still limited. Zack Lipton, a professor at Carnegie Mellon, has co-authored a paper showing one possible approach. It addresses the noise problem by predicting not stock prices, but the changes in company fundamentals—such as revenue or profit margins—that ultimately drive returns.
The adversarial nature of trading means that most developments remain shrouded in secrecy. That makes high-quality AI scientists hard to recruit. Scientists like to publish and collaborate. “We love discovering new things about markets and have a great community of people within the firm that we’re able to share results with, but unfortunately we can’t communicate them to a wider audience,” says Pete Muller, the founder of PDT Partners LLC and a pioneer in the field.
The prospect of searching for ghostly signals that eventually disappear can also dissuade some people from working in finance. “In my mind, a top researcher would need a two- to five-times salary multiple to completely forgo the ability to publish and make the lifestyle trade-offs necessary to work in finance,” Lipton says. Still, there’s the lure of a tough problem, combined with the chance to make serious money. “Using machines to beat the markets is a really difficult challenge,” says McAuliffe, whose résumé includes biological research and a stint at Amazon.com Inc. “But I don’t think it’s impossible.”
Fonte: aqui
Quais são as economias hiperinflacionárias?
Com o retorno do tratamento à inflação na contabilidade brasileira (vide aqui), resta saber em que situações o IAS 29 deve ser aplicado. O International Practices Task Force (IPTF) of the Centre for Audit Quality (CAQ) (via aqui) considerando os dados de diversos países, considera como status de economias "altamente inflacionárias", baseado nos critérios do IAS 29, a seguinte listagem:
Economias com inflação de três anos acima de 100%:
Angola
Argentina
República Democrática do Congo
Sudão do Sul
Sudão
Venezuela
Economias com inflação projetada de três anos maior que 100%:
República Islâmica do Irã
Iémen
Zimbábue
Economias com inflação de três anos superiores a 100% após um pico de inflação:
Suriname
Economias com inflação em três anos entre 70% e 100% ou com um aumento significativo (25% ou mais) da inflação durante o período atual
Libéria
Líbia
Economias com inflação de três anos acima de 100%:
Angola
Argentina
República Democrática do Congo
Sudão do Sul
Sudão
Venezuela
Economias com inflação projetada de três anos maior que 100%:
República Islâmica do Irã
Iémen
Zimbábue
Economias com inflação de três anos superiores a 100% após um pico de inflação:
Suriname
Economias com inflação em três anos entre 70% e 100% ou com um aumento significativo (25% ou mais) da inflação durante o período atual
Libéria
Líbia
Letras mais usadas na língua portuguesa
Eis uma listagem interessante e útil: as letras que mais aparecem na língua portuguesa. Usando um dicionário de português, a partir de 32 mil lemas (ou raiz de uma palavra). Eis a ordem, as
Interesse, sim. Mas útil? Quem nunca jogou "forca"?
07 julho 2019
Machine Learning em Finanças: prever é muito difícil
Machine Learning é algo antigo que é usado desde os anos 1980 no mercado financeiro. Apesar de toda evolução dos algoritmos nos últimos anos, a capacidade de previsão dessas ferramanentas é muita pequena.
Em suma: os mercados financeiros são inerentemente imprevisíveis. Existem vários motivos pelos quais é tão difícil prever. O engenheiro indiano Hardik Patel , que trabalha no Google e foi chefe num fundo quantitativo em nova york, destaca os principais motivos que dificultam isso.
1 º Distribuição dos dados: O futuro é distinto do passado. Esse é um problema comum quando se trata de aplicar o aprendizado de máquina a problemas do mundo real. Além de garantir que os conjuntos de teste e treino tenham distribuições semelhantes, também é preciso garantir que o modelo treinado seja usado apenas quando os dados futuros estiverem em conformidade com a distribuição do treinamento / validação.
2 º Amostra dos dados pequena: quando o conjunto de dados é bem pequeno, como no caso de estatísticas de desemprego fica bem difícil fazer previsões.
3 º Previsão em finanças é algo bem complexo: muitas coisas afetam os preços em diferentes escalas:
a) Em intervalos de alta frequência, o preço dos ativos é afetado pelos algoritmos de negociação;
b) Os preços de abertura e fechamento têm seus próprios padrões - tanto em ações quanto em futuros
c) Notícias e rumores são as forças motrizes quando se trata de horizontes de vários dias. Notícias específicas da empresa podem acontecer a qualquer momento sem aviso prévio. No entanto, a linha do tempo para alguns eventos é conhecida de antemão. O cronograma de resultados da empresa, bem como o calendário de dados econômicos, são conhecidos de antemão.
d) Valuation e os ciclos econômicos são importantes quando se trata de mudanças de preços em faixas de vários anos.
4º Outros problemas:
a) Previsões financeiras têm baixa acurácia;
b) Há muito ruído nos dados;
c)Dados financeiros têm sazonalidade;
d) Há eventos que nunca foram vistos pela máquina; os dados financeiros podem conter eventos totalmente diferentes do que estava disponível durante o treinamento do modelo.
e) um conjunto de dados financeiros pode consistir em preços de frequência mais altos, bem como dados econômicos de menor frequência.
Em suma: os mercados financeiros são inerentemente imprevisíveis. Existem vários motivos pelos quais é tão difícil prever. O engenheiro indiano Hardik Patel , que trabalha no Google e foi chefe num fundo quantitativo em nova york, destaca os principais motivos que dificultam isso.
1 º Distribuição dos dados: O futuro é distinto do passado. Esse é um problema comum quando se trata de aplicar o aprendizado de máquina a problemas do mundo real. Além de garantir que os conjuntos de teste e treino tenham distribuições semelhantes, também é preciso garantir que o modelo treinado seja usado apenas quando os dados futuros estiverem em conformidade com a distribuição do treinamento / validação.
2 º Amostra dos dados pequena: quando o conjunto de dados é bem pequeno, como no caso de estatísticas de desemprego fica bem difícil fazer previsões.
3 º Previsão em finanças é algo bem complexo: muitas coisas afetam os preços em diferentes escalas:
a) Em intervalos de alta frequência, o preço dos ativos é afetado pelos algoritmos de negociação;
b) Os preços de abertura e fechamento têm seus próprios padrões - tanto em ações quanto em futuros
c) Notícias e rumores são as forças motrizes quando se trata de horizontes de vários dias. Notícias específicas da empresa podem acontecer a qualquer momento sem aviso prévio. No entanto, a linha do tempo para alguns eventos é conhecida de antemão. O cronograma de resultados da empresa, bem como o calendário de dados econômicos, são conhecidos de antemão.
d) Valuation e os ciclos econômicos são importantes quando se trata de mudanças de preços em faixas de vários anos.
4º Outros problemas:
a) Previsões financeiras têm baixa acurácia;
b) Há muito ruído nos dados;
c)Dados financeiros têm sazonalidade;
d) Há eventos que nunca foram vistos pela máquina; os dados financeiros podem conter eventos totalmente diferentes do que estava disponível durante o treinamento do modelo.
e) um conjunto de dados financeiros pode consistir em preços de frequência mais altos, bem como dados econômicos de menor frequência.
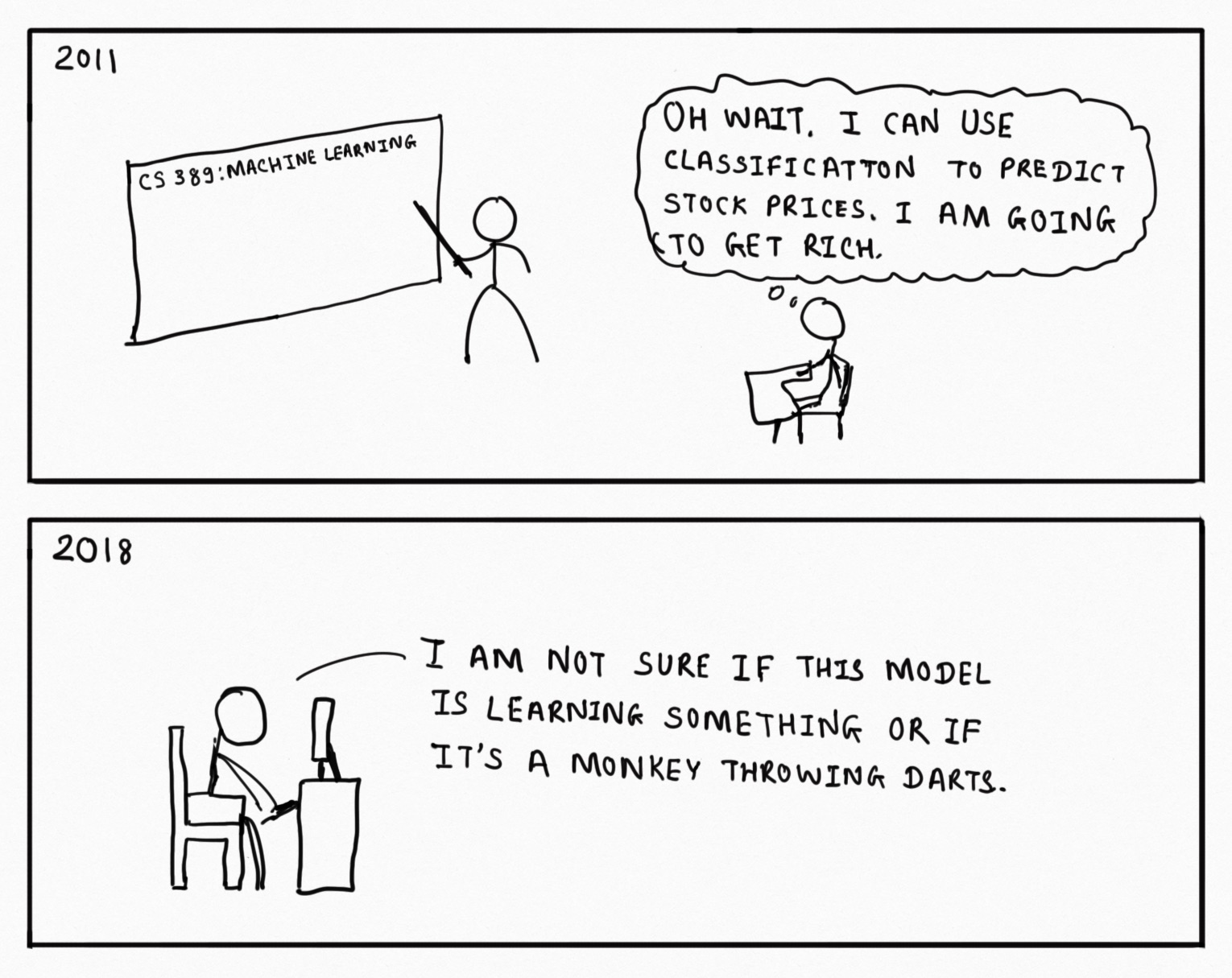
Domínio CPA
Os domínios da internet são regulados por uma entidade chamada ICANN. Esta é uma entidade sem fins lucrativos, vinculada ao governo dos Estados Unidos.
Agora, o AICPA, o Instituto de Contadores Públicos Certificados, com 330 mil membros, anunciou que o domínio CPA estará sob sua responsabilidade. Desde 2015 o presidente da entidade AICPA pressionava para obter esta responsabilidade. O presidente do AICPA estava preocupado com a possibilidade de alguém, que não fosse um contador público certificado, usasse e abusasse do domínio.
Isto pode ser um sinal de que o domínio ser usado especialmente por contadores públicos ou por pessoas que estariam dentro dos padrões e critérios definidos pelo AICPA. Entretanto, o Going Concern lembra que uma investigação em 68 mil domínios com "cpa" não achou evidências de problemas. O site, ironicamente, afirma:
Meaning there aren’t wild bands of rogue Internet trolls camping CPA domains for the purpose of diluting public trust or worse, offering professional services without a license. Go figure, Internet criminals have better things to do it seems.
Agora, o AICPA, o Instituto de Contadores Públicos Certificados, com 330 mil membros, anunciou que o domínio CPA estará sob sua responsabilidade. Desde 2015 o presidente da entidade AICPA pressionava para obter esta responsabilidade. O presidente do AICPA estava preocupado com a possibilidade de alguém, que não fosse um contador público certificado, usasse e abusasse do domínio.
Isto pode ser um sinal de que o domínio ser usado especialmente por contadores públicos ou por pessoas que estariam dentro dos padrões e critérios definidos pelo AICPA. Entretanto, o Going Concern lembra que uma investigação em 68 mil domínios com "cpa" não achou evidências de problemas. O site, ironicamente, afirma:
Meaning there aren’t wild bands of rogue Internet trolls camping CPA domains for the purpose of diluting public trust or worse, offering professional services without a license. Go figure, Internet criminals have better things to do it seems.
Assinar:
Postagens (Atom)